Predictive Technologies and AI in Education
Artificial Intelligence (AI) has made huge leaps in the past few years to become a common catch-all phrase referring to generative large-language models, machine learning, and even sometimes basic algorithms. Predictive technologies, like what ChatGPT is (or in other words, generative AI), has exploded in popularity – an anecdote from my own life is that I was walking around the UVic library looking for a spot, and almost all of the computers I saw had ChatGPT open. It’s used for all sorts of things – as it is a large language model, it is trained on so much data collected from the web to generate a best-fitting answer to a question. This is helpful because it can give you ideas for topics to write about, or even write it for you (a challenge that will be mentioned). A newer model is NotebookLLM by Google, specifically made for education/research purposes – you input your own training data and it will give an answer based on it. Overall, a significant area where AI is making an impact is on helping us learn new topics (Kim et al., 2022).
Benefits of AI in Education
It’s almost overwhelming the amount of options AI can present in the field of education, some undoubtedly positives. One of the main benefits that is presented is personalized learning to analyze student data and create tailored learning paths – this can also greatly benefit students with diverse learning needs or disabilities (Kim et al., 2022). In this way it can break down barriers and increase accessibility. Automation of tasks is also a good benefit, like administrative tasks that take up a teacher’s time – grading papers, and so on. AI chatbots can also act as language parters for students, allowing them to practice language skills in a fun and interactive way. I like to use AI to help with studying for exams, using bloom’s taxonomy. I find that a lot of teachers use the bloom’s taxonomy levels to base their exam questions and as a baseline for understanding concepts, so I will write into the chatbot to give me a practice test based on it.
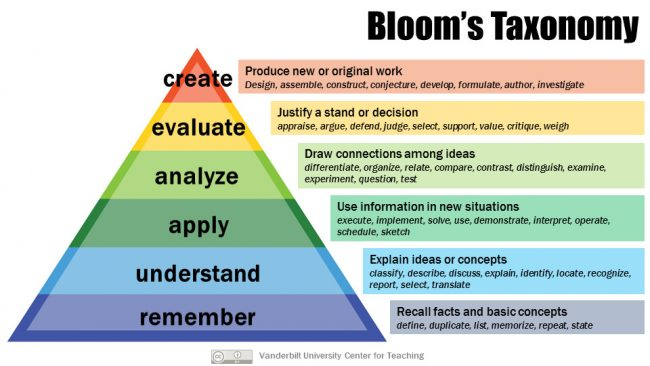
Challenges of AI in Education
AI also presents challenges in the education space. Barriers like high costs of implementation in some cases, technical expertise to know how, and internet access are most important, because without it, AI tools and platforms are inaccessible. Some schools, however, do not have the resources to implement AI to help. Privacy is also a big concern – large language models commonly used by students may be using student’s information to train. Also, the power being generated to train AI is huge, presenting a significant environmental concern and potentially raising power bills for schools. These factors combined create a complex landscape for AI adoption in education, demanding a thoughtful approach that balances potential benefits with practical and ethical considerations. To improve, we need more experts on educational-focused AI to make it more accessible and private for all.
Ethical Considerations of AI in Education
Data privacy is a key concern in using AI in education, as student data can be vulnerable to breaches or misuse. AI often relies on student data for training, raising questions about access and usage. Schools must enforce strong privacy measures and comply with laws like GDPR and FERPA. Bias in AI algorithms is another issue; if training data reflects societal biases, AI tools can reinforce discrimination, such as in biased college admissions decisions. The complexity of AI systems also creates transparency challenges, leading to mistrust. Institutions must explain how AI tools work, their limitations, and educate students on AI and privacy to ensure fair, ethical use.
Emerging Technologies Beyond AI:
The future of educational technology will likely focus on innovative platforms and engaging experiences. A major trend is short-form content, like YouTube Shorts, which makes learning more accessible and flexible. Coding tutorials already use this format, and other subjects could follow, offering quick, focused lessons for shorter attention spans. Learning analytics is another promising area, using data to personalize learning, track progress, and provide tailored recommendations. Combining short-form content with advanced analytics could create a dynamic learning ecosystem, but ethical issues like data privacy and bias must be addressed to ensure these tools are used responsibly.
References
Kim, J., Lee, H. and Cho, Y.H. (2022a) ‘Learning design to support student-AI collaboration: Perspectives of leading teachers for AI in Education’, Education and Information Technologies, 27(5), pp. 6069–6104. doi:10.1007/s10639-021-10831-6.